Predictive data analysis: the key to optimizing inventory management and anticipating customer demand
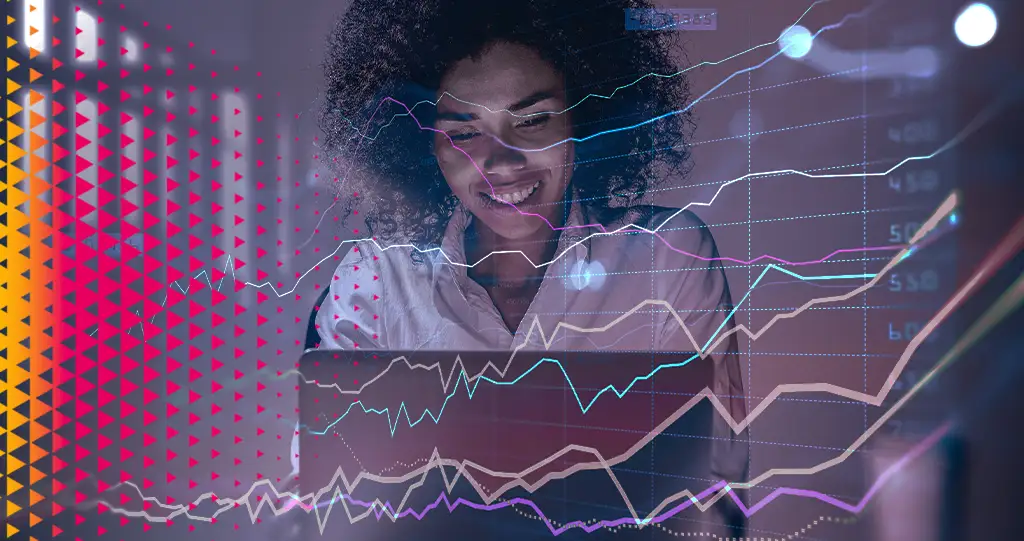
Managing stockouts, overstock, and replenishments are critical challenges that affect both profitability and customer satisfaction in the retail sector. Today, optimizing inventory requires advanced predictive analysis techniques that allow for accurate demand forecasting.
Learn how predictive analysis is revolutionizing inventory management.
Predicting customer demand
Importance of predictive data analysis
With customer interactions increasing across multiple channels, the data generated from these touchpoints becomes invaluable. Traditional forecasting methods based solely on sales history fall short in addressing contemporary buying behaviors. Predictive data analysis enhances accuracy by incorporating external factors.
Retailers are well aware that ineffective inventory management results in stockouts or overstock, negatively affecting both profitability and customer satisfaction. By leveraging predictive data analysis, retailers can anticipate demand and synchronize procurement accordingly.
Algorithms and tools for predictive analysis
Predictive analysis algorithms utilize historical data and trends to create accurate forecasts, employing artificial intelligence across various models:
- Simple moving average and exponential smoothing identify long-term trends;
- The ARIMA (AutoRegressive Integrated Moving Average) model captures seasonality to deliver more precise forecasts;
- Artificial neural networks uncover complex patterns, fine-tuning predictions.
Platforms like Orisha Commerce harness these models for advanced predictive analysis within unified commerce, allowing retailers to make data-driven inventory decisions and adjust supplies across all sales channels.
Impact of consumption trends on demand
Currently, 50% of French shoppers prefer a hybrid approach, switching between e-commerce and physical stores—a trend that’s on the rise. Additionally, 48% of consumers are more likely to buy when the offer is personalized, and a significant number now consider sustainability in their clothing purchases.
Retailers must evolve their strategies to meet these new challenges, offering seamless omnichannel experiences, personalized offerings, and addressing ethical concerns.
Effective replenishment strategies
Real-time stock level management
Unified data offers a comprehensive view of inventory across all channels, including stores, warehouses, e-commerce, and marketplaces. Traditional management often creates discrepancies between actual availability and forecasts. Real-time management, however, allows retailers to:
- Prevent stockouts;
- Reduce overstock and associated costs;
- Enhance the customer journey through click & collect and ship from store;
- Predict demand fluctuations.
Orisha Commerce’s unified commerce software synchronizes data across all channels in real-time, providing unified visibility and superior order orchestration. The Openbravo WMS module supports warehouse management on both cloud and mobile platforms.
Allocation techniques to avoid stockouts
Effective allocation means strategically distributing products among warehouses, stores, and online channels based on needs and logistical constraints. The aim is to prevent stockouts while optimizing storage and transportation costs.
Predictive demand allocation is driven by analyzing sales trends and buying behaviors, whereas dynamic allocation adjusts to ongoing sales, catering to the unique sales dynamics of each product type.
Explore the Orisha Commerce platform for dependable real-time data management!
Optimizing the reorder point and shipping times
The reorder point is the threshold for initiating a replenishment order to prevent stockouts. Predictive models dynamically adjust the reorder point according to trends.
While optimizing the reorder point is crucial, it’s equally important to fine-tune shipping times to ensure prompt product availability. Automation and real-time analysis expedite picking processes, prioritize urgent orders, and enhance carrier coordination.
Unified commerce and omnichannel
Integrating channels for a seamless customer experience
Unified commerce synchronizes all touchpoints to deliver a seamless customer experience. Unlike multichannel setups where each channel operates independently, omnichannel integrates customer data into a unified vision. This approach ensures a consistent shopping experience across all channels—store, e-commerce, marketplace, and mobile.
Openbravo SSMS offers a cutting-edge multi-store stock management solution, allowing retailers to monitor movements in real-time. Discover our solution today!
Role of technology in unified commerce
Unified commerce requires full integration of all sales channels. Platforms like Orisha Commerce bring together e-commerce, stores, marketplaces, logistics, and customer service into one cohesive ecosystem.
Utilizing artificial intelligence and predictive models, retailers can analyze unified data to forecast demand. For instance, luxury brand SMCP selected Openbravo for its cloud technology and unified commerce features, optimizing its omnichannel distribution. Explore our fashion-specific solutions to stay ahead of trends.
Benefits of an omnichannel approach to replenishment
An omnichannel strategy allows for dynamic replenishment management with a complete real-time overview. Inventory is distributed to stores and warehouses according to demand, with systems initiating orders as thresholds are met.
Measuring the success of forecasts and replenishment
Key performance indicators to track
To maintain optimal product availability, several key performance indicators should be tracked:
- Forecast error rate, which measures the variance between projected and actual demand. A high forecast error rate may indicate that the predictive models are not accurate enough;
- Service rate, which gauges the ability to fulfill customer demand based on available inventory. A high service rate suggests effective supply management, while a low rate might indicate potential customer dissatisfaction;
- Stock turnover rate, which reflects the frequency at which products are sold and replenished over a defined period. A high turnover rate denotes strong sales dynamics, whereas a low rate may point to overstock issues;
- Stockout rate, which shows the percentage of items out of stock compared to the total product catalog.
Adjustments based on post-sale analysis
Post-sale data enables companies to refine their strategies. By analyzing the gaps between forecasts and actual sales, businesses can adjust future calculations and optimize inventory levels based on product performance.
Examples of companies that improved product availability
Decathlon, a leading sports retailer, transformed its logistics management to better anticipate seasonal peaks. The company employed machine learning algorithms to analyze sales history and enhance forecast accuracy. Moreover, Decathlon streamlined its AI models for efficient deployment in production, significantly reducing stockouts.
Zara, known for its rapid collection turnover, often faced stockouts on popular items. To mitigate this, Zara implemented an agile replenishment model, blending frequent deliveries with centralized management. This approach successfully reduced stockouts and boosted sales.
Predictive data analysis enables companies to enhance product availability. Incorporating artificial intelligence into their strategies allows retailers to forecast customer demand fluctuations and optimize operations. Greater profitability and seamless customer experiences: it’s time to leverage the power of unified data!
Frequently asked questions
What methods are used for demand forecasting?
Forecasting methods fall into two categories:
- 1. Quantitative Methods
These rely on analyzing historical data through various models. Common techniques include:- Simple moving average and exponential smoothing for long-term forecasts;
- ARIMA (AutoRegressive Integrated Moving Average) model which accounts for seasonality to deliver precise forecasts;
- Artificial neural networks used in machine learning to detect complex patterns and provide real-time adjustments.
2. Qualitative Methods
Based on expert insights, these methods draw on market feedback, expert opinions, and external data to refine forecasts.
How is the replenishment level calculated?
Replenishment levels are determined by considering the reorder point—when an order must be placed to avoid stockouts:
Reorder point = (average demand x delivery lead time) + safety stock.
What are the advantages of accurate demand forecasting?
Accurate demand forecasting optimizes inventory management by preventing stockouts and overstock, ensuring customer satisfaction through product availability.
What is predictive analysis?
Predictive analysis uses historical data, statistical algorithms, and AI techniques to forecast future events. This involves identifying data patterns and trends. Methods like machine learning, statistical regressions, or neural networks help optimize decision-making across various sectors. Incorporating these tools into their strategy allows companies to increase agility and enhance customer experiences.
How does predictive analysis work?
Predictive analysis involves utilizing data for informed decision-making. Companies gather historical data from multiple channels and analyze it with advanced algorithms. These analyses identify patterns and provide precise forecasts, enabling real-time strategy adjustments.